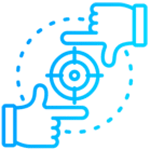
Client’s Challenge
Increasing Revenue and Client Reimbursement Rates
EnableComp wanted to refine its ability to recover underpaid zero balance claims, providing additional reimbursement faster for its clients.
EnableComp wanted to refine its ability to recover underpaid zero balance claims, providing additional reimbursement faster for its clients.
Through rigorous, exploratory analysis, our team developed a predictive model to identify which appeals would result in a successful outcome. We then crafted a final model that emphasized simplicity by maintaining a balance between the multiple parameters that impact an appeal outcome.
InfoWorks delivered a model that allows EnableComp to prioritize working appeals with the highest likelihood of a successful outcome. Specialized features allow prioritization of high-value claims and faster payments to cash-sensitive clients. InfoWorks built the model with foresight, allowing our client to scale with growth.
EnableComp is the nation’s leading workers’ compensation (WC) reimbursement expert who has processed over $1B+ in WC claims for over 500 health systems and hospitals nationwide. They recently partnered with InfoWorks to research additional analytics-based methods and predictive analytics tools to further enhance its appeal success rates on zero balance claims. A zero balance claim is a claim the member pays for in its entirety, typically because the cash price is lower than copay.
The complexity of WC and its variations in reimbursement because of state-specific fee schedules makes efficient, expedient claims processing and timely reimbursement a challenge. With its proprietary technology platform, Enforcer, EnableComp is a valuable resource to healthcare organizations who do not have the expertise on hand to navigate through the reimbursement process.
EnableComp wanted to provide additional reimbursement faster for its clients by recovering underpaid zero balance claims. Beginning with a thorough exploratory analysis, we developed a statistical model to accurately predict which appeals would result in a successful outcome. The overall goal was to inform decisions about the priority order for all underpaid claims, leading to the maximum amount of returns in the shortest time possible for clients.
Our team began by performing a survey of what attributes are known before the appeals process begins. This includes information about the patient, the procedures performed, the payer, supporting documentation, and the claim’s payment history. We then pulled together multiple years of data outlining past appeals and performed an advanced analytics analysis on each parameter to determine how much of a role it plays in predicting the outcome of an appeal.
Through this comprehensive analysis, we crafted a final predictive algorithm that emphasized simplicity by maintaining a balance between the multiple parameters that impact an appeal outcome. That simplicity appeared as a score that reveals the likelihood each appealed claim would be successful. Success was defined by both the probability that a reimbursement will be made by the payer and the amount of the reimbursement. Not only does the score account for multiple important factors, it simplifies it so that everyone in the organization can understand its impact and have the clarity needed for better decision making. The model was able to score the claims used in the test population with a high degree of accuracy.
With the new predictive model, EnableComp can prioritize appeals with the best chance of a successful outcome. In addition, the model: